Towards Robots that We can Trust
As robots make their way into our homes, the ability to predict how their actions affect the environment is a key skill that they need to possess to operate in safety and gain our trust.
In this work, we review the robotic pushing literature. While focusing on work concerned with predicting the motion of pushed objects, we also cover relevant applications of pushing for planning and control.
This paper is concerned with the evolution of forwards models (FMs) and their application in robotics. The reviewed papers are classified according to the type of approach implemented. We identify the following six classes.
1. Purely analytical: It is mostly seminal work drawn from classical mechanics.
2. Hybrid: It extends analytical approaches with data-driven methods. Whilst the interactions between objects are still represented analytically, some quantities of interest are estimated based on observations, e.g. the coefficients of friction.
3. Dynamic analysis: It integrates dynamics in the model.
4. Physics engines: It employs a physics engine as a “black box” to make predictions about the interactions.
5. Data-driven: It learns how to predict physical interaction from examples.
6. Deep learning: As the data-driven approaches, it learns how to construct an FM from examples. The
key insight is that the deep learning approaches are based on feature extraction.
Concluding remarks and further research perspectives are given at the end of the paper.
arXiv Preprint https://arxiv.org/pdf/1905.05138.pdf
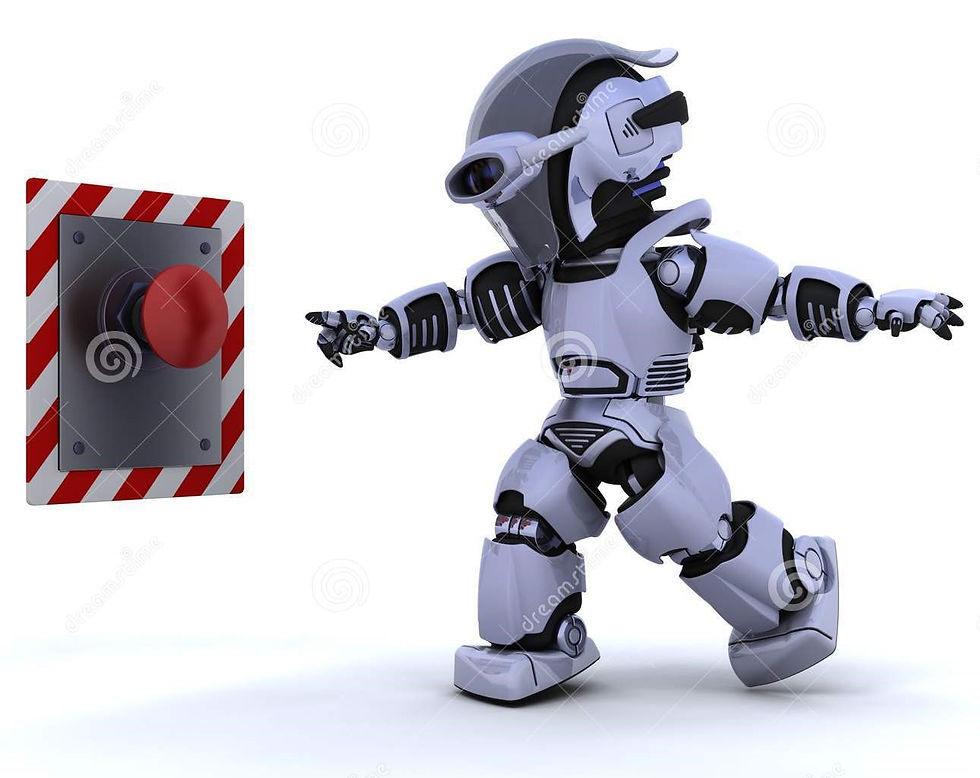